“
6Pages write-ups are some of the most comprehensive and insightful I’ve come across – they lay out a path to the future that businesses need to pay attention to.
— Head of Deloitte Pixel
“
At 500 Startups, we’ve found 6Pages briefs to be super helpful in staying smart on a wide range of key issues and shaping discussions with founders and partners.
— Thomas Jeng, Director of Innovation & Partnerships, 500 Startups
“
6Pages is a fantastic source for quickly gaining a deep understanding of a topic. I use their briefs for driving conversations with industry players.
— Associate Investment Director, Cambridge Associates
Read by
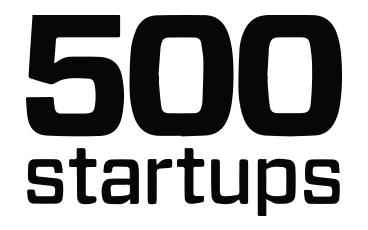
Used at top MBA programs including
May 2 2025
15 min read
1. The flurry of activity around robotaxis
- A lot has been happening in the world of robotaxis. Since California approved Waymo to operate 24/7 paid robotaxi services in SF (Aug 2023) and Cruise foundered following a widely reported accident (Oct 2023), Waymo has been gaining rapid traction. Last week, Waymo reported 250K+ paid robotaxi rides every week across the US, in Phoenix, SF Bay Area/Silicon Valley, Los Angeles, and Austin (with Uber). This is up 5x from a year ago and up 25% from Feb 2025. Waymo has become the “biggest part” of Alphabet’s “Other Bets” portfolio, which saw $450M in revenue in Q1 2025. Next for Waymo are rollouts in Atlanta (with Uber) this summer and then Miami and Washington DC in 2026, as well as expanding its partnerships with Uber, automakers, and fleet-management vendors.
- While Waymo is the clear leader now, it won’t have the field to itself forever. Last week, Tesla began tests of a Supervised Full Self-Driving (FSD) ride-hailing service with employees in California and Austin. (Tesla was granted a very limited permit in Mar 2025 in California, and Texas has relatively lax rules regarding driverless vehicles.) These services will use Tesla’s existing Model 3 and Model Y vehicles. In Austin, it plans to launch a paid, Unsupervised FSD robotaxi service using its Model Y vehicle, starting in Jun 2025 with 10-20 vehicles. In addition to California and Austin, Musk says Tesla is on track to be in “many other cities” by the end of 2025.
- Tesla has already begun prototype builds of its Cybercab – an efficient 2-person EV without steering wheel or pedals unveiled in Oct 2024. It hopes to ramp up to volume production in 2026 and eventually reach production of 2M+ Cybercabs per year. The Cybercab will utilize Tesla’s “unboxed” manufacturing process, which CEO Elon Musk describes as akin to a high-speed, highly automated consumer-electronics production line, with a single-piece large casting per vehicle. Eventually, a Cybercab could roll off its line every 5 seconds. On the Q1 2025 earnings call, Tesla execs noted that work to ready Cybercab production lines in its existing Gigafactory Texas (Austin) facility is already underway this year. Tesla’s Cybercab and other specialized AVs (without equipment like steering wheels, pedals, and mirrors) will be accelerated by the National Highway Traffic Safety Administration (NHTSA)’s recent rule revisions exempting AVs from certain standards.
- Waymo has not fully nailed down its long-term business model. Alphabet/Google CEO Sundar Pichai suggested on Alphabet’s recent Q1 2025 earnings call that Waymo’s business model – whether it be licensing, autonomous vehicle, standalone ride-sharing, delivery, or something else – could be different by geography. He even hinted at “future optionality around personal ownership” of self-driving vehicles. Tesla is also aiming for the “personal AV” market and hoping to be able to turn on full autonomy in existing vehicles through an over-the-air update, starting this year and into 2026.
- Partnerships are a key avenue for Waymo in scaling up its operations. On Alphabet’s Q1 2025 earnings call, Pichai said Waymo is “building up a network of partners.” Uber is its most prominent partner, participating in Waymo rollouts in Austin (where Waymo already represents 20% of Uber rides) and Atlanta. Uber is managing these autonomous fleets through its partially-owned affiliate Avomo, although Waymo retains some responsibilities. (Uber has been on a tear with its AV partnerships lately, including recent deals with Volkswagen, May Mobility, and Momenta.) Waymo also has other partners, such as Moove for fleet operations/maintenance in Phoenix and Miami, as well as a number of auto OEMs.
- While “future optionality around personal ownership” might suggest this being far out, Waymo recently signed a deal with Toyota to explore self-driving personal cars. Autonomous vehicles (AV) as personal cars would be heavily constrained by their current cost, which – at an estimated $150K-200K each – would far exceed the median conventional vehicle. There would also likely be subscription fees associated with software development, maintenance, and connectivity. In the near term, this suggests a relatively small market consisting of very wealthy individuals, shared community vehicles, businesses looking to provide transport for customers and employees, and perhaps parents who need to drive cadres of highly scheduled children to extracurriculars. (AVs may start looking reasonable relative to the ongoing variable costs of drivers and nannies.) There’s also the possibility that prices could drop rapidly as AVs enter scale production, opening up this market.
- Musk is known for his willingness to break the rules in pursuit of its ambitions. For instance, the Grok model family from his AI arm xAI – which acquired X (formerly Twitter) in Mar 2025 – has been noted to demonstrate fewer guardrails than its rivals, offering “edgy” humor and anti-woke commentary. When it comes to robotaxis, however, consumers are likely to steer towards the safer, more conservative option, all things being equal. Waymo reports its vehicles are involved “81% fewer injury-causing crashes” vs. human drivers.
- Things may not be equal though. Waymo’s rivals can slash their prices to try to compete, for instance. While this may not be a sustainable model for some players, Tesla could have an advantage in being vertically integrated and already producing its existing vehicles at scale. Musk has suggested that a Tesla built at scale might cost about 20-25% of a Waymo, which has an “expensive sensor suite.” Tesla vehicles only use cameras paired with AI, an unusual decision for a player with autonomous ambitions. (Nearly every other player uses lidar sensors combined with cameras.) Musk has said he expects the price of a Cybercab to eventually be sub–$30K.
- It should be noted that Waymo’s vehicle cost is also coming down – in large part because its 6th-gen Driver system will be built on a Chinese-made base vehicle (Geely Zeekr electric minivan) with fewer cameras and sensors. While Biden and Trump’s tariffs are impacting the economics of imported vehicles, Waymo has avenues it could go down. It could negotiate a bulk deal with Geely akin to what Amazon did with Rivian, bringing the per-vehicle cost down. It could also ask Geely – which owns Volvo and Polestar and has US plants – to manufacture its vehicles in the US. Finally, Waymo could expand its OEM partnerships – it is already integrating the 6th-gen Driver into Hyundai’s Ioniq 5 and now plans to include Toyota vehicles in its ride-hailing fleet.
- A key question is how long will Waymo keep the lead. If we consider the public cloud – another highly capital-intensive business – Amazon invented the market with AWS and had a 4-year head start before Microsoft entered with Azure. To this day, AWS remains the leader in the public cloud, despite the size of the industry and how lucrative it has become.
- It took Waymo 16 years to get here (from 2009), backed by Google’s wallet and a team of the brightest autonomous-driving minds. With respect to the Levels of Automation (0-6), Waymo reached Level 3 in 2012 and Level 4 by 2017. (No AV to date has reached Level 5, which requires operating in all conditions and on all roadways.) For comparison, Tesla – which has a decade of Musk’s self-driving promises in its rearview – has been working on its Autopilot system for 12 years (2013), and only introduced Autopilot 10 years ago (2015) and FSD 4 years ago (2021). Tesla reached Level 2 autonomy in 2015, and has been nearing Level 3 (“eyes off”) for some time. In short, AV development is hard and not linear. Even China, which has been pushing on AVs hard, recently began cracking down on overhyped marketing language after a deadly crash. (The crackdown impacts Tesla as well as Chinese players.)
- The volume of autonomous miles driven matters. Musk alluded to the challenge of iterating and improving upon models when you have to drive vehicles enough miles to get an intervention, which might take place “every 10,000 miles.” With Waymo with tens of millions of autonomous miles under its belt and likely driving another 1.5M+ miles per week, its algorithms are certainly continuing to improve.
- Waymo is reportedly testing and mapping in 10 new cities this year, including Las Vegas, San Diego, and Tokyo. It is aiming for improved “generalizability” of its technology across localities, which could accelerate the rollout. (Tesla is also taking a generalized approach, although Musk recently said this may be subject to a “localized parameter set.”) Waymo is furthermore working to knock down barriers to adoption, focusing on areas like airport access (e.g. SFO), freeway driving, and winter driving. It is also continuing to focus on improving safety. If Waymo is not just better and safer but also getting better and safer faster, its long-term leadership may already be sealed.
- Of course, much depends on whether Google is broken up and can afford to continue to invest. (“Other Bets,” where Waymo sits, lost $1.2B in Q1 2025.) Google has lost major antitrust cases related to search and adtech over the past year. In the search case, the Justice Dept is asking for a spinout of Chrome and for Google to be required to share search histories and other data with rivals. According to Pichai, the proposed remedies would jeopardize user privacy and security, make it “unviable to invest in R&D,” and result in “many unintended consequences.” Chrome’s head has said that Chrome’s interdependencies mean it may not even be possible to carve it out. In the more recent adtech case, the DOJ is asking for a sell-off of Google’s sell-side adtech tools. Notably, both judges were appointed by Democrat presidents (Obama and Clinton, respectively) and it is now Trump’s DOJ that is seeking Google’s break-up.
Related Content:
- Jan 10 2025 (3 Shifts): Uber steers toward autonomous driving
- Aug 23 2024 (3 Shifts): Waymo’s 100K+ paid robotaxi rides every week
2. OpenAI and AI players inch towards ads
- AI players have been inching towards ads over the past 4-6 months. Earlier this week, OpenAI revealed that shopping is being rolled out this week in ChatGPT. Users – both signed-in and logged-out (including Plus, Pro, and Free users) – may see product cards and/or a shopping pane (akin to Google Shopping) pop up based on their query. (The feature is currently available in GPT-4o and 4o-mini.) Buy buttons next to products will take users to merchants’ websites to complete transactions. OpenAI says the results are organic and independently selected rather than sponsored.
- ChatGPT will provide product recommendations based on open-ended queries like “gifts for someone who loves cooking” or “best noise-cancelling headphones under $200” or “I’m looking to buy costumes for my two dogs.” ChatGPT then takes what it knows about user preferences (via its memory feature and custom instructions), and combines them with sources like structured product metadata (e.g. price, description), online reviews, and Reddit. In addition to considering the specific criteria provided by the user, ChatGPT may also consider general factors like price, ratings, ease of use, and its own safety standards.
- Merchants looking to be shown in ChatGPT search results need to make sure they have not blocked OpenAI’s search-focused bot OAI-SearchBot in their robots.txt file. OpenAI is exploring a way for merchants to submit their product feeds directly, which would enable “more accurate, up-to-date listings.” For the time being, merchants can complete an interest form to be notified when submissions open. Merchants can also start tracking their referral traffic coming from ChatGPT through popular analytics tools like Google Analytics. (ChatGPT adds “utm_source=chatgpt.com” to all of its referral URLs.)
- With ChatGPT on the receiving end of 1B searches per week, it seems inevitable that OpenAI would move in this direction. Google has shown just how lucrative search can be when paired with ecommerce. OpenAI CEO Sam Altman recently changed his tune on advertising, expressing an openness to ads if they were “tasteful.” While OpenAI insists that its results are organic, there are other ways to make money from advertising than sponsored results or altering the recommendations/rankings. For instance, merchants could bid on the ability to be the seller linked to a product (akin to Amazon’s Buy Box or Featured Offer). OpenAI could also take an affiliate-marketing commission (e.g. a 2% fee) on any traffic it refers that turns into a sale.
- Shopping is also likely a good use case for generative AI, given that the query, criteria, and results can all be relatively structured. It can be served well with lower-compute models like GPT-4o and 4o-mini. Shopping is also a use case where back-and-forth conversation (vs. keywords as with Google Shopping) can lead to better results. OpenAI’s new feature already has its critics, however – some are saying that OpenAI’s results are too wordy, don’t have enough visuals, are not well-sourced, lack the rawness of end-user reviews, and may miss some options. These are issues that OpenAI can readily resolve though, and it’s still early days.
- OpenAI has been making changes of late that, in retrospect, seem a bit oriented towards building an ads/ecommerce-adjacent business. These changes include bolstering its Free tier (to lure in more eyeballs) and launching a memory feature (to facilitate personalization). Some of its newer products such as Operator and Deep Research are also relevant for ecommerce/advertising.
- OpenAI isn’t the only AI player moving towards ads. Perplexity began introducing ads to its “answer engine” platform in mid-Nov 2024, starting in the US. These ads are included among the follow-up questions below or beside Perplexity’s responses, and are labeled as “sponsored.” Answers will not be written or edited by sponsors, and will continue to be generated by Perplexity. Separately, Perplexity introduced shopping features for paid users that allow users to buy with one click. Merchants can enroll in a program to provide Perplexity with more complete information so their products are more likely to be recommended.
- Obviously, all this is a threat to Google, which generates 87% of US search referral traffic today and has the #1 browser. Google is already facing challenges with the loss of two major antitrust cases (see above), which could result in remedies like the spinout of Chrome and forced sharing of user histories with rivals. Google CEO Sundar Pichai has said that, with this data, “It would be trivial [for rivals] to reverse engineer and effectively build Google search from the outside.” Not coincidentally, both Perplexity and OpenAI have expressed interest in buying Chrome, which could be worth as much as $50B by one estimate. Perplexity is already working on its own browser to support its personalization efforts. Its goal is to “collect data on everything users do outside of its own app.”
- It’s a threat to Amazon as well, given its role as the internet’s one-stop ecommerce shop. Amazon has long been the place where users looking for a specific item or who have a particular need would go for research and purchase. Now, OpenAI has a rumored integration with Amazon rival Shopify in the works, which could boost Shopify’s profile and offer a fat referral pipeline to its distributed network of merchant sites.
- It’s also a threat to affiliate marketing sites, including those owned by large media companies (e.g. NYTimes’ Wirecutter). If OpenAI is synthesizing content that it uses to supplant the original content site, that’s fodder for a likely lawsuit. (NYTimes is already engaged in a copyright lawsuit with OpenAI.) Revenue-sharing is one option for AI players if their sources are concentrated in a few non-permissioned sites. On the other hand, a player like OpenAI may be able to get what it needs from user-generated forums like Reddit (which has a deal with OpenAI) and voluntary product-feed submissions from merchants, without giving away anything.
- Much of what we take for granted about today’s internet is shaped by ads-based business models like Google’s. A shift in the business model towards gen AI players has the potential to reshape the big-tech landscape, how content is created, and how individuals meet their information needs. Advertising could become more interleaved into a contextual conversation, and perhaps more relevant for the user. If AI players can build trust among users that they will provide accurate, unbiased answers – even as they pursue an ads-based business model – it could change how users view advertising. Inevitably, as the stakes get higher, there will be more people and firms looking to capture a piece of the pie (and game the new system).
Related Content:
- Apr 18 2025 (3 Shifts): The growth of AI big tech and $200-per-month subscriptions
- Dec 6 2024 (3 Shifts): Perplexity and other gen AI players eye advertising
3. Interpretability and how AI models think
- While artificial general intelligence (AGI) has long been the North Star for many AI researchers, AI models today are still probabilistic systems that find patterns in their training data and make predictions based on those patterns. A growing field called “mechanistic interpretability” is offering insight into how AI models think. Researchers are finding that how models think appears to be more akin to a collection of rules-of-thumb or “bag of heuristics” than human-like “world models” and reasoning.
- What is the difference between bags of heuristics and world models? One researcher defines world models as capturing causal structure, being abstract/compressed (and algorithmically efficient), and relevant to the agent’s tasks.
- A recent research paper investigating how AI models do arithmetic – a highly algorithmic task – found that large language models (LLMs) like Llama 3 don’t use generalizable algorithms or even memorization. Instead, they rely on shallow, task-specific patterns – a "bag of heuristics" – to approximate reasoning. Models might have a different set of rules for multiplying numbers in a specific range (e.g. from 200 to 210) than another range – obviously a less efficient approach than using a generalizable deterministic rule. Even with a very large bag of heuristics that can be selected from and layered on top of each other, models still can’t perform relatively basic arithmetic problems with perfect 100% accuracy.
- The remarkable fluency of some models can be very convincing. However, they are often brittle when encountering new situations, collapsing on out-of-distribution examples. Even models that can solve arithmetic problems with 90%+ accuracy do worse when the format of the input is changed. A model providing turn-by-turn directions in NYC might nose-dive in performance when 1% of the roads are blocked (in large part because its “mental map” is a yarnball of heuristics that includes impossible routes).
- This suggests that models are faking coherence by layering heuristics, but without necessarily understanding the meaning of the content or being able to reason logically from that meaning. Rather than viewing LLMs as flawed versions of human-like agents, they may be closer to evolutionary hacks – accumulations of useful tricks with no internal consistency. While this doesn’t make them less useful, it does complicate robustness, safety, and explainability.
- Anthropic CEO Dario Amodei recently added urgency to this debate, describing in a blog post his company’s vision of making AI models less of a black box – “reliably detect[ing] most model problems” – by 2027. Amodei argues that interpretability has lagged behind AI progress. Anthropic is aiming to use mechanistic interpretability to trace the pathways for how models think and choose at the neuron/circuit level – to understand why, for instance, a model that performs better on some tasks might also hallucinate more. AI models can also show “jagged intelligence” – impressively smart in some ways and extremely dumb in others.
- Amodei believes that mapping the circuits responsible for reasoning and decisions will enable deeper control over AI behaviors (and failures). Eventually, this could be like a brain scan or “AI MRI”, although it might take 5-10 years to get there. Amodei stresses the importance of interpretability research for safety and alignment, especially as models take on greater responsibilities as autonomous agents. In Amodei’s words, “We can’t stop the bus, but we can steer it.”
- Understanding how models think could help AI developers, for instance, systematically block jailbreaks or divulgence of dangerous knowledge. It could also help researchers detect the emergence of undesirable behaviors like deception, alignment-faking, and power-seeking – recognizing when a model is “unhealthy” or in distress.
- Reasoning models are “thinking out loud,” creating the impression of greater transparency. However, model interpretability is far from straightforward. While there are some interpretable single neurons (akin to neurons in vision models) that might represent specific words and concepts, most are not immediately interpretable. Anthropic call this phenomenon “superposition,” which means concepts are layered and entangled in a way that allows a model to express more concepts but can result in high opacity for a human examining it.
- Interpretability researchers are using neuroscience-like techniques such as probing individual neurons and mapping activation clusters. The discovery of a technique (from signals processing) called “sparse autoencoders” (SAE) is helping researchers find combinations of neurons (“features”) that correspond to human-understandable concepts (e.g. “genres of music that express discontent”) Sparse autoencoders are starting to be open-sourced, giving researchers tools to understand AI models’ features. Anthropic is also applying “autointerpretability” – using AI to analyze AI for features and identify what they mean in human terms. It found, for instance, 30M features in the medium-sized Claude 3 Sonnet – although this is just a fraction of the 1B+ features in a small model.
- It’s possible that AI models are just in a phase of toddlerhood where their bags of heuristics are currently disorganized – i.e. this current state may not be permanent. Some argue that models are already doing some form of generalized reasoning but just not as well as a human. At some point, the bags of heuristics may become synthesized and compressed into something like an emergent worldview.
- Amodei has put forward some suggestions on how to advance interpretability, including encouraging other AI players to work on interpretability and advocating for light-touch regulation. (Anthropic has begun investing in interpretability startups.) Amodei is also advocating for export controls to provide a buffer so interpretability has time to catch up.
- It’s not clear whether export controls will hamper or accelerate the progress of AI models. There’s an argument to be made that AI models have landed on “bags of heuristics” as a local maxima, and that compute constraints could accelerate their progress towards more algorithmically efficient world models. Biological constraints – the brain’s energy usage and need for food – have shaped humans into what we are, relying on a “mixture of experts” with both probabilistic and (more efficient) deterministic pathways. In the same way, compute constraints could meaningfully shape AI. Some believe China’s progress in AI (e.g. DeepSeek) can be attributed to prior export controls, although China has been working on AI and chips for some time. At the very least, how Chinese players have pursued AI has been shaped by expectations of scarcity.
- Yann LeCun has pointed to the limits of language: “A system trained on language alone will never approximate human intelligence, even if trained from now until the heat death of the universe.” According to LeCun, AI models understand language only in a shallow way – lacking the full-bodied knowledge that humans gain from living and acting in the real world. AI models lack the biological hormone-based feedback mechanism that mediates the human experience – such as pleasure (dopamine), stress (cortisol), trust (oxytocin), and risk-taking (testosterone). AI benchmarks reward outcomes but not principle or process, leading to a narrow focus on improving outcomes.
- If models function through bags of heuristics rather than generalized reasoning, it challenges the idea that they can ever be truly aligned to human values. After all, the application of fundamental human values requires reasoning from first principles. This has implications for trust, safety, and deployment in high-stakes settings such as medical choices, legal judgments, and military/defense contexts. Until models are interpretable, AI development will continue to be a live path-dependent game of trial and error.
Related Content:
- Feb 14 2025 (3 Shifts): “Deep research” tools everywhere
- Feb 7 2025 (3 Shifts): Distillation and AI economics
Become an All-Access Member to read the full brief here
All-Access Members get unlimited access to the full 6Pages Repository of766 market shifts.
Become a Member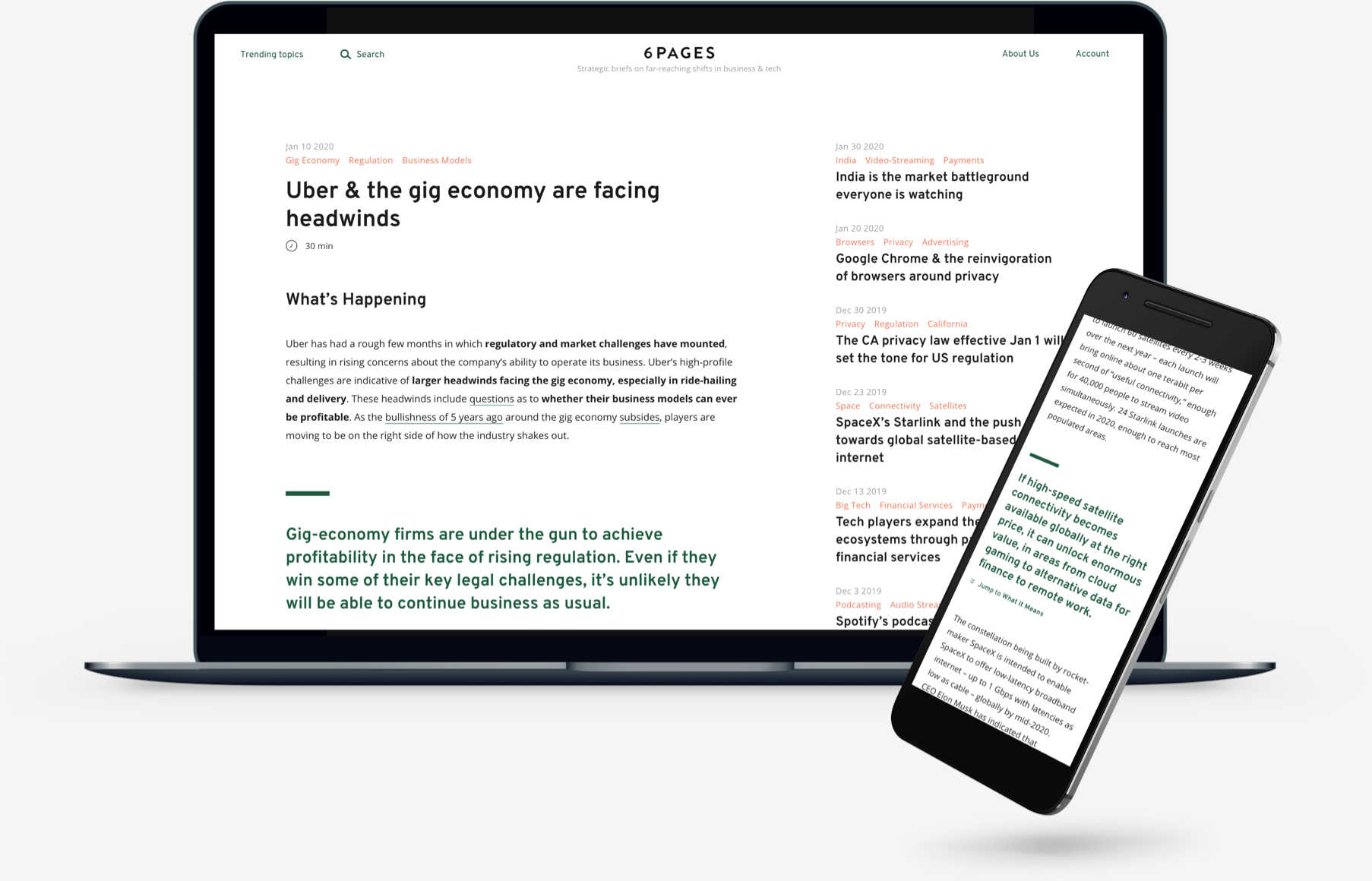
Already a Member?Log In
Disclosure: Contributors have financial interests in Meta, Microsoft, Alphabet, Uber, OpenAI, and Perplexity. Amazon, Google, and OpenAI are vendors of 6Pages.
Have a comment about this brief or a topic you'd like to see us cover? Send us a note at tips@6pages.com.
All Briefs
Get unlimited access to all our briefs.
Make better and faster decisions with context on far-reaching shifts.
Become a Member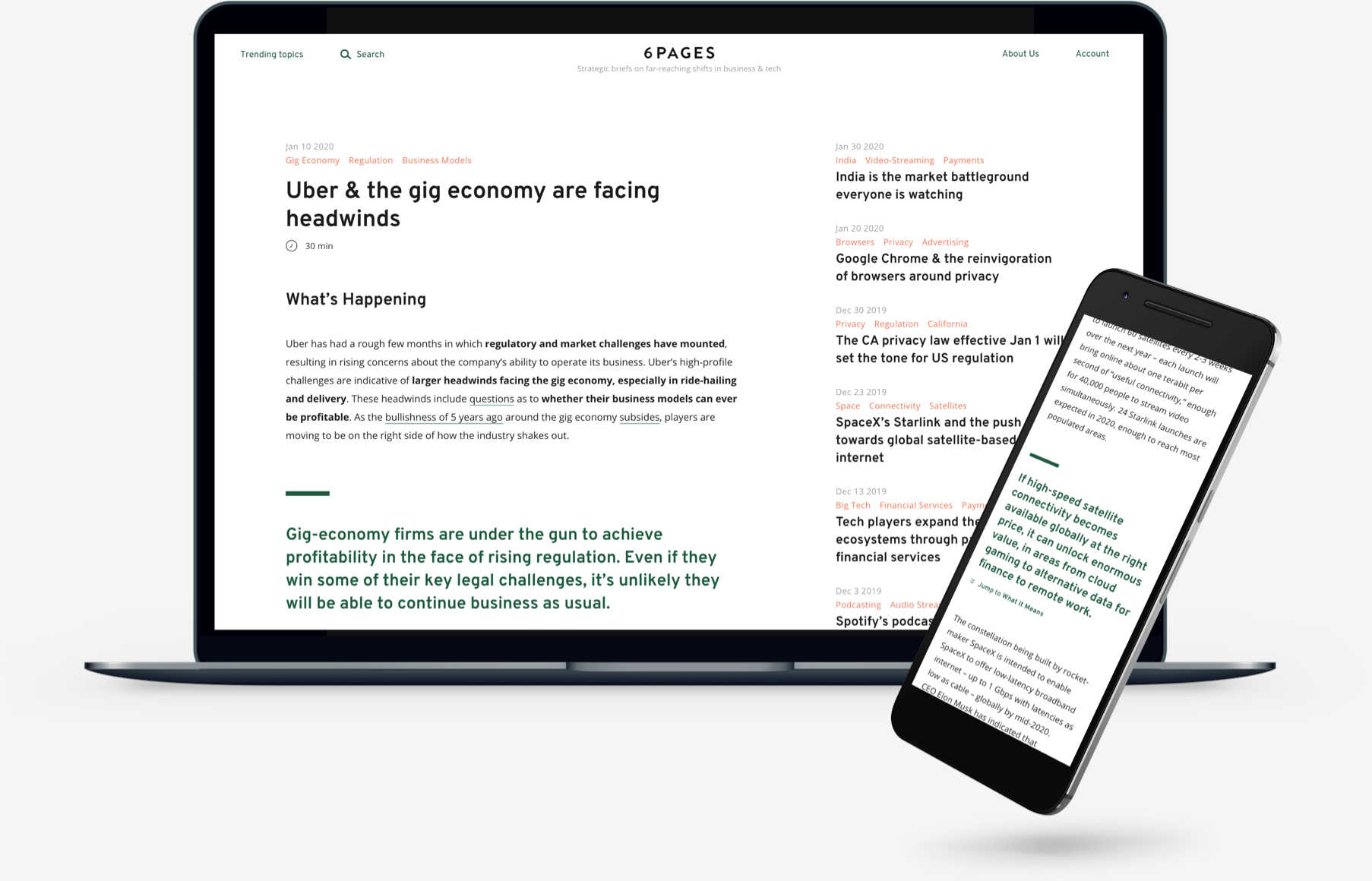
Already a Member?Log In
Get unlimited access to all our briefs.
Make better and faster decisions with context on what’s changing now.
Become a Member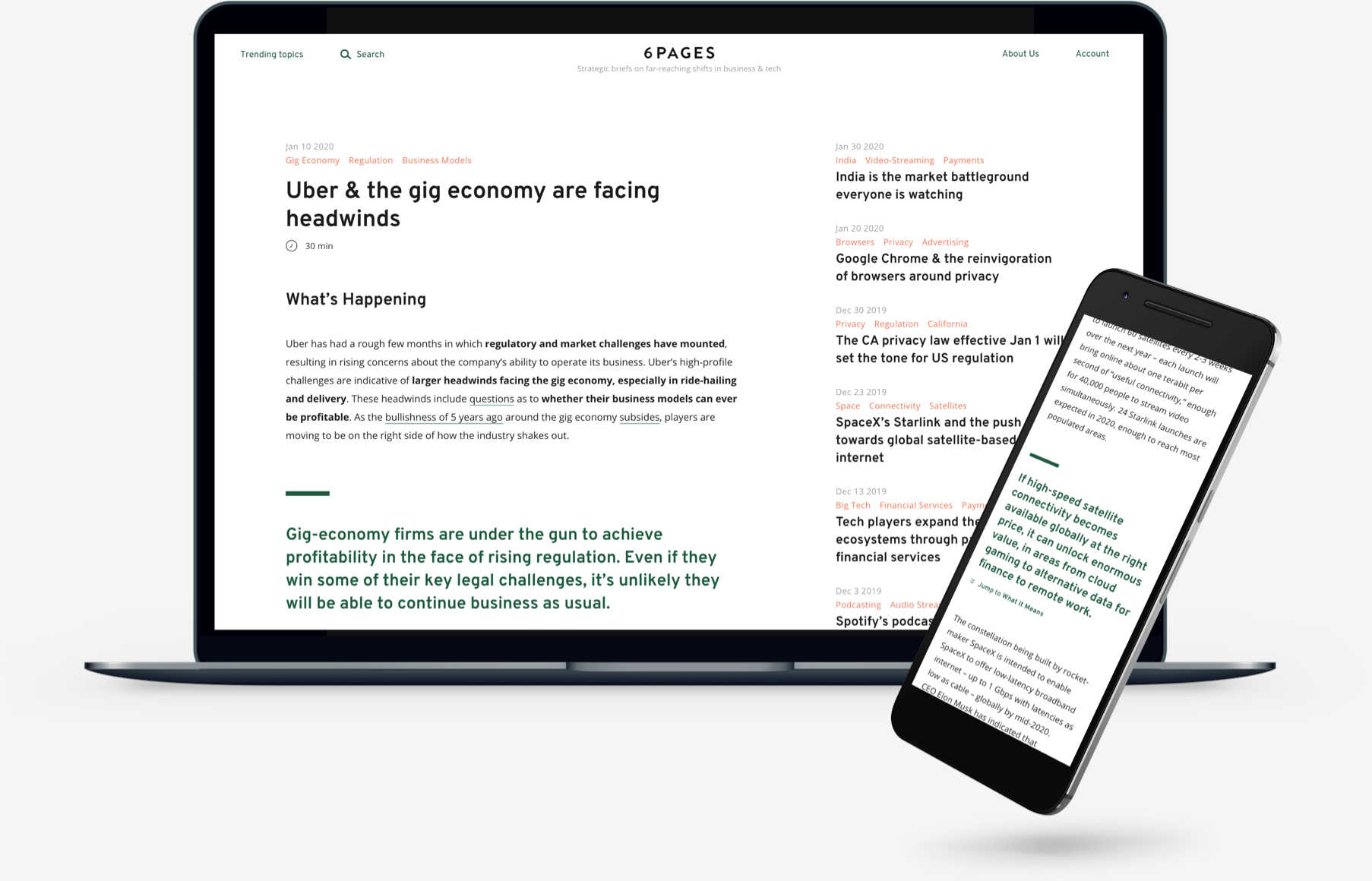
Already a Member?Log In